Unlocking Transparency with XAI770K: The Future of Explainable AI
Artificial Intelligence is transforming industries, but traditional AI models often lack transparency. Many businesses struggle to understand how AI makes decisions. This creates trust issues, especially in critical sectors like healthcare, finance, and law.
Explainable Artificial Intelligence (XAI) is a groundbreaking approach designed to solve this problem. It focuses on AI transparency, accuracy, and adaptability. Unlike conventional black-box AI models, XAI provides clear insights into how it reaches conclusions.
Interpretable Machine Learning is becoming essential for ethical AI adoption. Governments and organizations demand AI systems that are transparent and accountable. Explainable AI is a step forward in making AI more understandable and trustworthy.
One of the biggest challenges in AI is balancing accuracy and interpretability. Traditional models often prioritize performance over clarity. AI Model Interpretability bridges this gap by maintaining high precision while keeping its decision-making process transparent.
Businesses benefit from AI models they can trust. When stakeholders understand AI predictions, they can make better decisions. Transparent AI Models ensure that AI-driven insights are not just powerful but also explainable.
This model is versatile and can be applied across different industries. From AI in Healthcare to AI in Finance and AI in Cybersecurity, it helps professionals make smarter choices. Its adaptability makes it a valuable tool for various AI applications.
Regulations around AI are evolving, making AI Accountability a priority. Companies that fail to adopt explainable AI risk non-compliance with future laws. AI Model Transparency aligns with ethical standards and regulatory requirements.
The future of AI depends on models that people can trust. AI Decision-Making Processes represent a shift towards responsible AI innovation. AI Decision Interpretability sets a new benchmark for AI systems that prioritize both performance and clarity.
Aspect | Key Facts | Figures/Comments |
---|
Explainable AI (XAI) | Provides transparent insights into AI decision-making; resolves trust issues with traditional black-box models. | Emphasis on clarity, no specific numeric figures provided. |
Interpretable Machine Learning | Enhances ethical AI adoption by ensuring AI outputs are understandable; critical in sectors such as healthcare, finance, and law. | Promotes accountability and improved stakeholder trust. |
Accuracy & Interpretability | Strikes a balance between high precision and clear, explainable outcomes; maintains performance without sacrificing transparency. | Accuracy remains comparable to conventional models while adding clarity. |
Adaptability & Versatility | Can be applied across various industries (healthcare, finance, cybersecurity, retail) by adjusting to unique data challenges and business needs. | A versatile tool that adapts to different datasets and applications. |
Scalability | Designed to handle large datasets efficiently; suitable for real-time data analysis and growth in data volume without compromising performance. | Ideal for companies with expanding data requirements. |
Security & Compliance | Incorporates AI ethics, bias mitigation, and secure decision-making processes; helps organizations meet regulatory requirements and maintain ethical standards. | Critical for regulatory compliance in sensitive sectors. |
Real-World Applications | Supports practical use cases such as fraud detection, medical diagnostics, personalized retail recommendations, and government policy analysis. | Demonstrates broad industry impact with actionable AI-driven insights. |
AI Decision-Making Processes | Clarifies how decisions are made by AI models, enhancing accountability and trust; supports informed decision-making by stakeholders. | Helps bridge the gap between automated insights and human understanding. |
Regulatory & Ethical Standards | Ensures AI systems are compliant with evolving regulations; emphasizes fairness, transparency, and ethical use in AI-driven decisions. | Mitigates risks associated with non-compliance and unethical AI practices. |
Industry Impact | Empowers industries to leverage AI for smarter decision-making while ensuring transparency and fairness; leads to better outcomes in finance, healthcare, retail, etc. | Aligns with the growing demand for ethical, transparent AI solutions. |
Core Features and Capabilities of Explainable AI
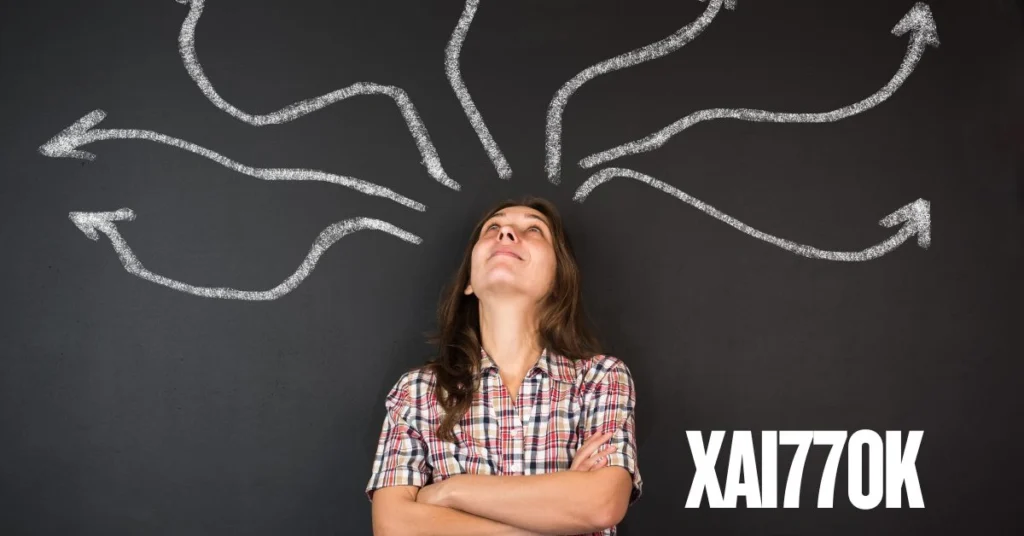
This AI system is designed to be transparent, accurate, and adaptable. It stands out from conventional machine learning models by offering better insights into its decision-making process. These key features make it highly valuable across industries.
Explainability: Transparent AI Models for Decision-Making
Most machine learning models function like black boxes, making it hard to understand their logic. AI Transparency explains its reasoning step by step. It enables users to trust and validate AI-driven conclusions.
Industries such as AI in Finance and AI in Healthcare demand AI that justifies its outputs. AI Model Transparency provides detailed insights, ensuring compliance with industry regulations. AI Decision Transparency improves trust and reliability.
Accuracy: High Precision in AI Decision Support Systems
AI is only useful if it delivers consistent and reliable results. AI Model Explainability is designed to maintain high levels of precision. It processes vast amounts of data while ensuring minimal errors.
Unlike traditional models, AI Ethics does not trade accuracy for transparency. AI Decision Interpretability ensures that explainability and performance go hand in hand. This makes it ideal for applications that require both precision and clarity.
Adaptability: Versatile AI Decision-Making Processes Across Industries
Every industry has unique data challenges. AI Bias Mitigation is flexible and can be applied across various sectors, including AI in Healthcare, AI in Finance, and AI in Cybersecurity. It learns and adjusts to different datasets, making it highly versatile.
Organizations do not need separate AI models for different tasks. AI Model Accountability can be fine-tuned to meet specific business needs. Its adaptability allows companies to optimize AI applications efficiently.
Scalability: Handling Large Datasets Efficiently with AI Decision Transparency
As businesses grow, so do their data requirements. AI Transparency Tools are designed to scale effortlessly with increasing data volumes. AI in Security processes large datasets rapidly without compromising performance.
Companies dealing with real-time data analysis can rely on AI Bias Reduction. Whether analyzing customer behavior or detecting fraud, this AI solution handles complex tasks seamlessly.
Security & Compliance: AI Ethics and Meeting Ethical Standards
AI should be both powerful and ethical. AI in Financial Services is built with fairness and security in mind. It follows strict guidelines to minimize biases and ensure responsible decision-making.
With increasing AI regulations, compliance is more important than ever. AI Model Fairness aligns with global standards, making it a safe and trustworthy AI solution. Businesses can adopt it confidently while ensuring ethical AI use.
Real-World Applications and Industry Impact
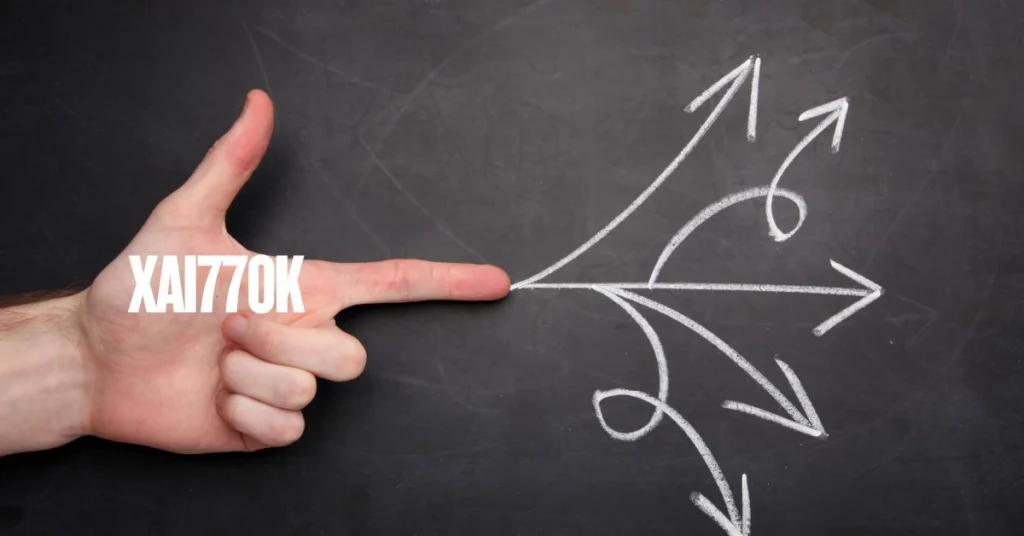
AI-powered systems are designed for real-world applications. Their ability to provide transparent and accurate insights makes them useful across multiple industries. Businesses, governments, and researchers can all benefit from their capabilities.
Business & Finance: Smarter AI in Financial Services
Financial institutions use AI for fraud detection, risk analysis, and investment predictions. AI Model Interpretability enhances these processes by making AI-generated insights explainable. It allows banks and investors to rely on data-driven recommendations with confidence.
Regulatory requirements demand that AI models justify their outputs. AI Decision Transparency ensures compliance while improving accuracy in financial forecasting. AI in Finance reduces risks and strengthens decision-making processes.
Healthcare: AI in Medical Diagnostics for Better Patient Outcomes
Medical professionals use AI for diagnostics, treatment plans, and drug discovery. AI in Healthcare enhances these applications by providing clear explanations for medical predictions. Healthcare providers can understand why certain treatments are recommended.
Transparency in medical AI is essential for patient trust. AI Model Ethics ensures that AI-driven healthcare solutions are ethical and reliable. AI Model Fairness helps bridge the gap between automation and human expertise.
Retail & E-Commerce: Enhancing Customer Experience with AI Transparency
Retailers rely on AI for personalized recommendations, pricing strategies, and demand forecasting. AI Transparency Tools make these processes more transparent and accurate. Businesses and customers alike can understand why specific products or offers are suggested.
Supply chain management also benefits from AI Decision Support Systems. Companies can anticipate demand trends and optimize inventory levels. AI Bias Reduction leads to better efficiency and cost savings.
Government & Policy Making: AI Model Accountability for Public Decisions
Governments use AI for policy analysis, legal decisions, and administrative processes. AI in Security ensures transparency in decision-making, allowing policymakers to trust AI-driven insights.
Legal and law enforcement sectors benefit from AI systems that prevent biases. AI in Security ensures fair and ethical AI applications in governance. AI Model Transparency reduces risks associated with opaque decision-making.
Conclusion
Artificial intelligence is reshaping industries, but for it to be widely adopted, transparency is essential. Explainable AI offers a promising solution to the issues surrounding traditional AI models, allowing businesses and individuals to understand how AI makes decisions. By providing clear, interpretable models, this technology ensures accuracy, accountability, and adaptability across various sectors. Whether in healthcare, finance, or government, organizations benefit from AI systems they can trust. With evolving regulations and an increasing demand for ethical standards, explainable AI represents a future where intelligent systems are both powerful and comprehensible.
What is Explainable AI?
Explainable AI refers to artificial intelligence systems that provide transparent insights into their decision-making processes. Unlike traditional “black-box” models, explainable AI ensures that users can understand and trust AI-driven conclusions.
Why is Explainable AI important?
Explainable AI is important because it builds trust and accountability in AI systems. It addresses transparency issues, making it easier to understand why certain decisions or predictions are made, which is especially crucial in critical sectors like healthcare, finance, and law.
How does Explainable AI differ from traditional AI models?
Traditional AI models often work as black boxes, making it hard for users to understand how they reach their conclusions. In contrast, Explainable AI offers clear insights into the decision-making process, ensuring that both accuracy and transparency are maintained.
What are the key benefits of Explainable AI?
The main benefits include improved trust, transparency, better decision-making, reduced risks of biases, and enhanced compliance with regulatory standards. It allows organizations to understand and validate the decisions made by AI systems, fostering greater acceptance and accountability.
Can Explainable AI be applied across different industries?
Yes, Explainable AI is highly adaptable and can be applied in various industries, including healthcare, finance, retail, and government. Its ability to provide clarity in decision-making makes it a versatile tool in improving processes across different sectors.
How does Explainable AI ensure compliance with regulations?
Explainable AI helps organizations meet regulatory requirements by providing clear explanations for decisions. This transparency makes it easier for businesses to demonstrate that their AI systems are compliant with industry regulations, ensuring ethical and lawful practices.
What challenges exist with Explainable AI?
One of the main challenges is balancing the need for high precision with the requirement for transparency. Some models may struggle to provide clear insights without compromising on accuracy. However, advancements in AI research continue to address this gap, making Explainable AI more efficient and accessible.
How does Explainable AI improve decision-making in businesses?
By offering clear explanations for AI-generated insights, businesses can make more informed decisions. Stakeholders can better understand the rationale behind predictions and outcomes, leading to improved decision-making processes across various functions, such as risk analysis, investment strategies, and customer engagement.